UN-Habitat Dialogue with Scientists: Interview 1 with Professor Qiang Yang
Professor Qiang Yang is Chair Professor at the Department of Computer Science and Engineering at Hong Kong University of Science and Technology (HKUST), and Chief AI Officer, WeBank.
Professor Yang, tell us a bit about yourself. Where did you study? What scientific fields do you focus on?
I grew up in Beijing, and my university was Peking University where I studied physics, Astrophysics. I was fascinated by astronomy. I pursued my bachelor’s degree to study how the solar system works, how the stars and galaxies work. Then, after my graduation, I got a scholarship in the University of Maryland. And then I went to a PhD in astrophysics.
But during my PhD, I observed that the world was undergoing a revolution, that is the computer revolution. People were using data science to discover knowledge rather than using artificial means to discover knowledge. So, at Maryland I took some courses in computer science and AI, and I then formally transferred to study artificial intelligence. I got my PhD in AI. It was the early 90s, late 80s. Then I moved to the University of Waterloo in Canada to teach AI and thereafter ended up staying for 13 years in Canada doing research in artificial intelligence.
Around that time AI was undergoing a mind shift: going from logic and human-oriented to a data-oriented approach. And the birth of machine learning was just starting at the beginning of the 90s, which I was fortunate to be part of. I devoted all my time studying AI and machine learning. At the same time, Asia was also on the rise and, observing that, I came to Hong Kong and was very impressed and decided to settle in Hong Kong as a professor. That was in 2001. I taught at Hong Kong University of Science and Technology as a faculty member and continued my research in machine learning up until today. Now I work in the intersection of AI and finance. My place of work now, WeBank, uses AI to offer online financial services and technique services. Now with the internet and AI, we can provide the same level of services to everyone, not just the “VIPs”.
We know you just were just at the World Urban Forum in Abu Dhabi, how did you feel about the event?
I think it was really eye opening for me. People were concerned about the future of humankind and human society in respect to urban issues. As we know, more and more people are moving into cities across the world. It's because of a variety of reasons like resources and education. It's this natural phenomenon that attracts our special attention. At the conference, I saw people concerned about urban issues. And increasingly, I feel that AI and technology can be important in achieving these goals.
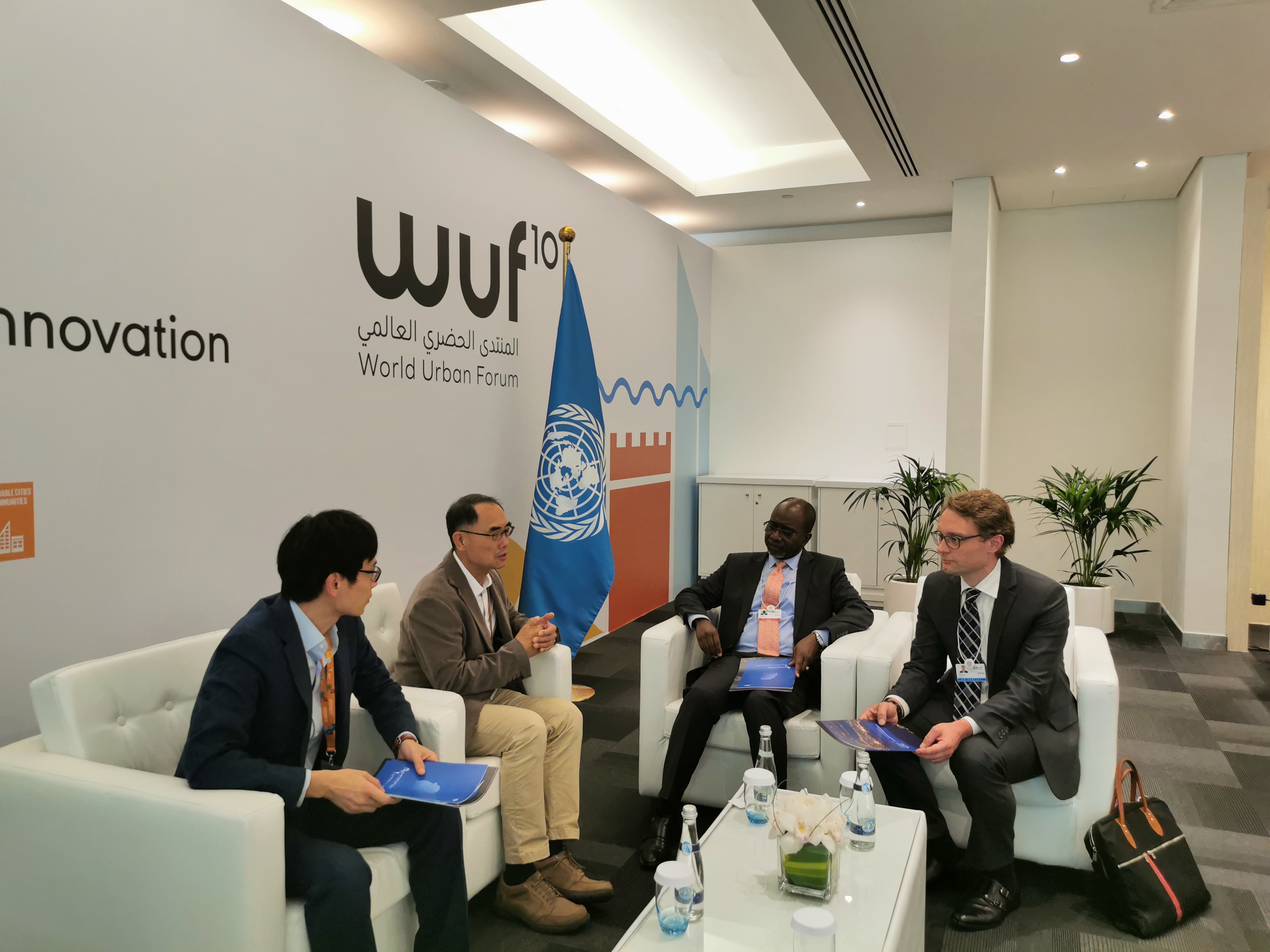
Your AI department does a lot of work with satellite imagery in cities. Could you explain this a bit more?
With advancement of rocket and satellite technology, satellite imaging is becoming more affordable. To the point that if you want to know key information about a wide geographic area, ordinary businesses can’t afford that. For example, in foreign aid, let's say there’s a bad flood, the traditional way of investigating would be to send humans; but that process is slow, dangerous, and inaccurate. Today, with low flying satellites, we can almost immediately assess the level of damage. So, if there is an insurance claim, we can find out whether the claim is genuine or fraudulent. We can design new insurance products that are more useful to victims. This is just one example. Satellite imaging can help assess city's traffic systems, design a better traffic system, and improve emergency services. We can assess housing demand in different regions of a city and whether the housing construction is enough. And all this information can be garnered from a frequently updated bird's eye view.
When we talk about AI and Big Data, everyone asks questions of security concerns. How does WeBank account for this security concern, and is your solution compliant with General Data Protection Regulation (GDPR)?
There are many issues around data security, and one issue is users’ privacy, which is becoming more acute and very much in the media. A popular case is the recent data leakage from big companies like Facebook, and even Google was fined in Europe for a violation of GDPR and other related privacy laws. On the other hand, each individual company, even a big company, usually has limited data. For example, consider a hospital that wants to use images for real-time diagnosis of cancer. There must be enough medical images in order to build such a model. But the problem is, usually with a single hospital, the amount of data is limited. So, multiple hospitals need to collaborate. But they are troubled by privacy concerns. It's a double-edged sword to collaborate. They collaborate and risk revealing patient's data without permission, or they don't collaborate and don't have enough data. We need a solution that can satisfy both ends. Both protect privacy and promote collaboration.
To put it simply, to build an AI and machine learning model across multiple data owners, you need to satisfy that no private data is leaked, and data is used in full knowledge of the multiple data owners. This calls for an international standard that makes it easy for many partners to work together. We at WeBank have been doing all this. We are leading IEEE standards in federated learning. We are donating an open source software to the Linux Foundation to allow anybody to use it for free and build their federated system, and we are working with several organisations, like hospitals, banks, and financial institutions. So far, the results are particularly good for the future of cities. The issue of privacy protection and responsible use of AI will become better only via technical experts and policy makers going hand in hand.
We would like you to talk a little bit more on federated learning. The public is more familiar with machine learning, deep learning, and natural language processing. But federated learning is a new concept to the public. How is this positioned in the AI world or in the AI spectrum?
The current surge of AI is mainly due to the development of a technology called machine learning or deep learning. What machine learning does is if you have accumulated a huge amount of data, such as images, then you can feed these images into an algorithm. What you would call a deep learning algorithm. The output of that algorithm is a decision system, which is like a collection of rules, in which one can look at a new picture and decide who that person is or what the object is. There’s a critical process which is known as “training the model” to build the collection of rules. So, anyone who wants to build a machine learning system first must get a large amount of data. Your model is just as good as your data. We tend to explain this using the concept of a farmer who is grazing sheep. He wants the sheep to grow up and be valuable, and, therefore, must feed the sheep a lot of grass. The sheep is the model in this story, and the grass is the data. So, of course, if you have a way to accumulate and refresh your grass and data, you can keep the sheep growing. But let's say you have a shortage of grass. Now the farmer must change his way of operation. The farmer will have to take the sheep walking through various locations where there's new grass. So, it’s the same way you must take the model through different data stores so that the model can grow. And in this process, if the model moves around, and not the data, then we can protect the privacy of the data. No data travels out, it is the model that goes along. This is the idea we are trying to tell, to take a model that we want to build with maybe two or three parties and relate the model to each party’s data. And when we go through this process, we make sure that no party can decipher or peek into other people's data. The model can benefit from each party’s data while protecting the respective parties.
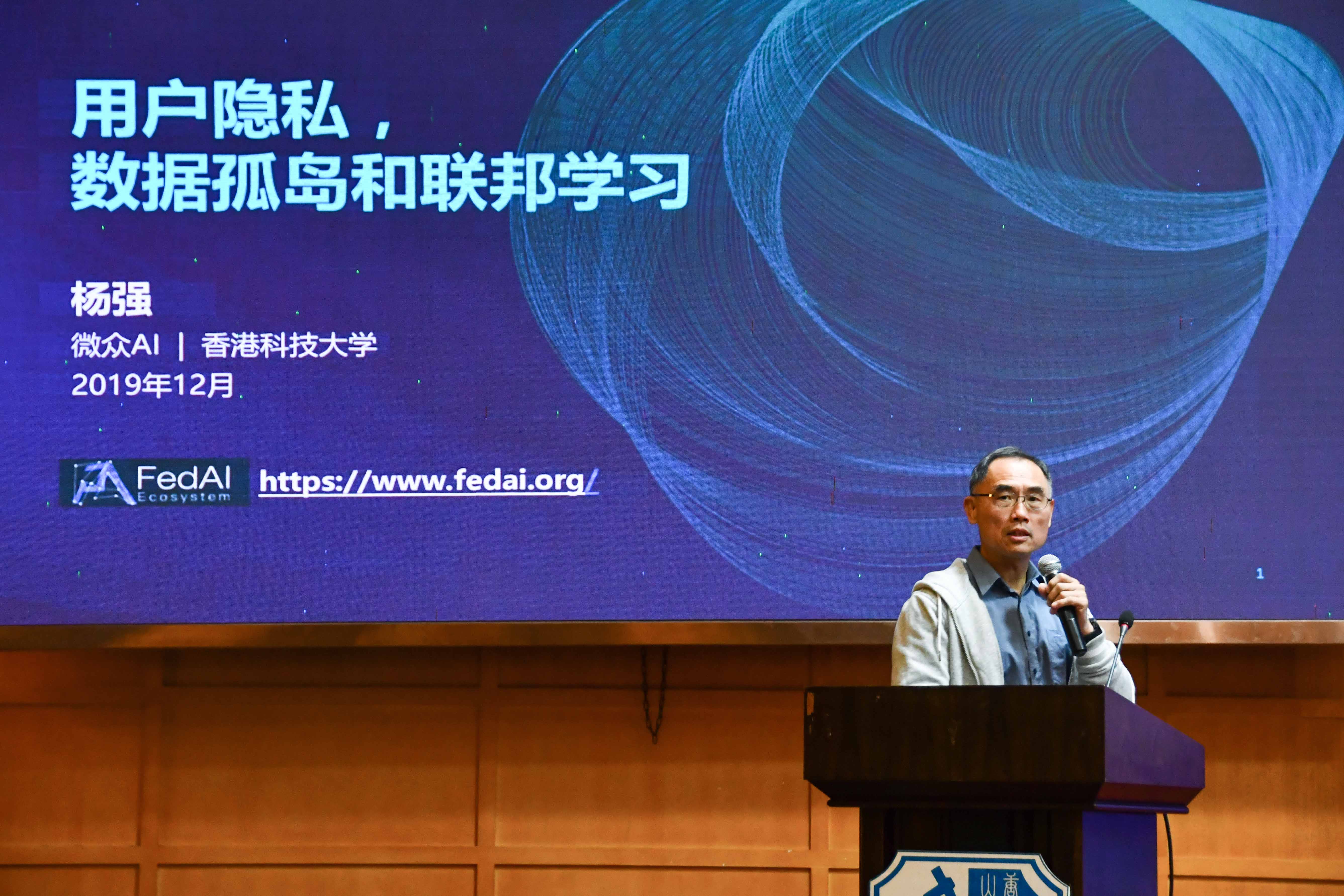
You spoke at the tenth World Urban Forum on Environmental, Social and Governance Investing, also known as ESG investing. Can you explain to us how the concept of ESG investing is becoming more prominent in China?
For a company to be profitable, we should not only look at the current state of business but consider from a long-term perspective. A long-term view which is not just profitability, but also considers sustainability - how the company treats the environment, how the company handles its social responsibility, and how well the company is governed. ESG is correlated with the long-term wellbeing of businesses. We can set ESG as a goal, but how do we find out from thousands of companies and cities whether each company or each city is adhering to ESG? How do we compare them and see which one is the more progressive? How do we lower the cost of doing so? These are the issues we must address with technology.
For example, if you have a satellite that scans the sky in a certain region and suppose there are some environmental polluters, such as coal burning without much treatment. From the satellite image, we can see the smoke, we can assess the pollution levels, and we can give a prediction on the ESG compliance. Just another example of how this enterprise can be very useful to the public and to government. There is an ESG index in the process of being created. In China, there are a couple of large fund companies that have started to use ESG as a ranking mechanism for financial products. WeBank, we are the first to use AI and satellites to generate ESG scores for companies, and we expect this to be utilised for a new style of funds in China.
On the issue of health, we would like to with WHO to establish a measurement metric to evaluate city’s responsiveness to epidemic outbreaks. Do you think AI and technology could contribute to the rapid assessment process?
That's a very current issue, a very pressing issue. In fact, using satellites, we can gain a lot of information about human traffics. For example, by cars, by buses, by trains, and even airplanes between any two regions, and we can give early warnings for a spread of a virus through observation. Such data has already been used to warn people of the upcoming flu spread. For example, Google has used Google search as a predictor for winter flus. It has been successful in warning cities through the number of searches of the flu from one city to the other. The searches were a strong indicator of the number of flu cases between cities. Likewise, we can check the number of hospitals, the density of schools, and the density of human habitats and work habits. All of these are strong indicators that AI can be used to serve public health.
Do you think there are any concrete actions that WeBank and UN-Habitat could deliver together?
I think there are many things we can do together. It's quite exciting, especially after talking to many colleagues at UN. For example, we can use big data, AI, and satellite technology to get a better idea of how cities develop. One area that we have a lot of experience is in inclusive finance. Using AI and big data to help underserved populations in their financial wellbeing: generating credit scores for small and medium sized businesses, risk assessments, generating insurance policies, creating marketing tools, and delivering long-term services to those in need. Another possible area of collaboration is in using satellites to better assess the economic status of different regions of a city, and thus allowing city decision makers to be more accurate and targeted with their decisions.